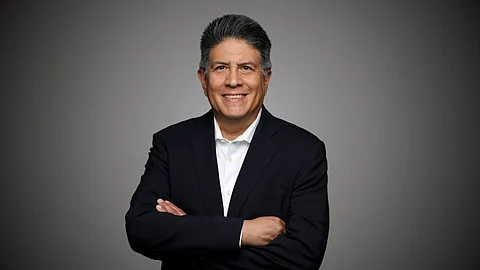
Over the past three years, accurately forecasting demand has become more challenging due to external factors such as the COVID-19 pandemic, global trade wars, and skyrocketing inflation.
As manufacturers grapple with additional disruptive elements like climate change, geopolitical tensions, and the need to realign supply chains, it is clear that returning to the previous state of "normal" is unlikely. This evolving landscape requires a reevaluation of forecasting strategies to build resilience and efficiency in the face of ongoing uncertainties.
Old forecasting models, which rely heavily on deterministic forecasting and historical data to predict future demand, have proven insufficient in today's volatile environment. Evidence shows that these models often fail to account for rapid changes and unexpected events.
According to a 2021 study by Logistics-as-a-Service platform e2open, the rate of planning errors is alarmingly high, with more than half (54%) of manufacturers' forecasts being wrong. This significant margin of error underscores the need for more sophisticated, adaptable forecasting methods that can handle the complexities of modern supply chains.
In this interview, demand forecasting expert Ed Coronado shares insights on the benefits and challenges of demand forecasting, as well as how modern methods and AI are helping overcome these challenges and improve accuracy. Coronado is an experienced data, analytics, and AI expert who has worked with global organizations to leverage the power of data, analytics, and AI to improve forecasts. His expertise has led to improved production, optimized inventory management, enhanced profitability, and happier customers.
Demand forecasting is nothing new for businesses. Accurate demand forecasting is like an operational crystal ball, helping businesses anticipate future needs and align everything from production to staffing, to inventory levels, and more. For example, a retailer can use forecasts to ensure they have enough stock of popular items during peak shopping seasons, minimizing the risk of stockouts. This minimizes costs associated with excess inventory or stockouts and allows companies to respond swiftly to changes in consumer behavior, economic conditions, and competitive landscapes.
Accurate demand forecasting relies on numerous technical and organizational factors, including a clear alignment with the business goals, access to diverse datasets, and an understanding of market fluctuations.
However, the biggest challenges for most organizations are their approach to sourcing and integrating quality data and the introduction of external data. Traditional modeling approaches, such as a deterministic approach, often rely on simple statistics and historical data, which struggle to address factors like seasonal variations, economic shifts, or sudden changes in consumer preferences.
For instance, a sudden economic downturn can drastically change consumer spending patterns, which traditional models might not predict. They are also difficult to adapt and scale to today's complex markets and high-volume datasets.
A modern forecasting program must incorporate several key components to ensure accuracy and adaptability. First, having the right data is essential. Forecasts need to move beyond just sales history and include a comprehensive view of both internal and external factors. This means integrating sales, customer, vendor, and inventory data with external factors like climate, macroeconomic conditions, and supply chain dynamics.
Second, stochastic optimization is a game-changer. Unlike deterministic approaches that assume a single outcome, stochastic optimization acknowledges the variability in future demand. This allows businesses to better understand and evaluate a full range of possible outcomes, enhancing your ability to make informed decisions.
Third, leverage AI and machine learning to process vast and diverse data and extract complex insights and patterns that are too intricate for manual approaches. This leads to more accurate and reliable forecasts and, at the same time, allows for scenario planning.
Fourth, adopting a holistic viewpoint is vital. Viewing demand as a single value ignores the inherent fluctuations of today's global market. By considering demand as a probability distribution, companies can set their risk tolerance and plan accordingly, which helps mitigate risks and seize opportunities.
Finally, having clear business goals is imperative. Organizations that optimize their forecasting toward specific goals — such as reducing costs, managing inventory, optimizing price, optimizing supply chain, or preventing stockouts — are better able to tailor their activities and leverage forecast results to support their desired financial outcomes. This strategic alignment ensures that forecasting efforts directly contribute to achieving business objectives.
A lot of the specifics will depend on the industry, the organization's technical foundation, and their previous experience with demand forecasting. However, here are a few general best practices to consider when getting started:
Define objectives and scope: Clearly define program objectives and focus points (For example, are you focusing on inventory management, production planning, or sales forecasting? What's the benefit to the company as well as the customer?), and determine the full scope of your forecasts, including the level of detail needed for decision-making.
Collect and prepare data: Identify your relevant internal and external data sources, cleanse and validate that data to ensure quality, and centralize it in a common repository or data warehouse for accessibility and analysis.
Select forecast models: Consider your product characteristics, market dynamics, and accuracy needs, as well as the benefits and drawbacks of statistical and machine learning models. Determine your needs and assess model performance using historical data to select the most suitable approach.
Implement and calibrate models: Deploy your chosen model(s) using appropriate tools and fine-tune parameters to fit specific data patterns and business context. As a best practice, avoid overfitting the data to the model, as it can potentially skew the results and limit the ability of the model.
Generate and evaluate forecasts: Regularly produce forecasts for defined scopes and timeframes and continuously monitor their levels of accuracy and potential biases. Adjust models as necessary to better fit needs and increase accuracy.
Integrate and automate forecasting: Integrate forecasting with ERPs, CRMs, inventory systems, and other technologies for seamless data flow and automate data collection, updates, and forecasting to streamline operations.
Monitor and improve: Continuously monitor performance and refine models, update data sources, and incorporate feedback to enhance accuracy and drive adoption.
About the Author:
Ed Coronado is an experienced leader in data, analytics, and AI. In his role at Wavicle Data Solutions, Coronado has helped companies design and implement technical solutions, including demand forecasting, to solve complex business challenges. If you are interested in learning more about how a modern approach to demand forecasting can help you make better operational decisions, reduce risk, or increase profits, please reach out to Ed on LinkedIn or at info@wavicledata.com.