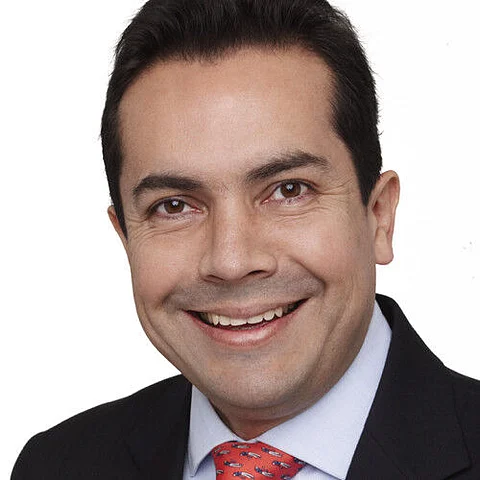
(US and Canada) In conversation with Sanjay Acharya, VP Strategy & Growth, HiLabs Inc., Luis Velandia, Chief Data Officer, Best Doctors Insurance, talks about the need for the right data governance models and the impact of bad data quality on businesses.
He says that not a single data governance model fits all organizations. The decentralized approach is best for small individual businesses and users maintaining their master data. It is simpler to maintain and faster to set up. However, if it is not managed properly, users can see inconsistencies in the data.
Centralized governance is based on multiple business units centralizing the setting up and maintenance of master data on a central organization based on consumer requests. It works better for large or medium organizations but calls for complex requirements like larger systems and master data to be distributed to different systems.
The hybrid model has a centralized body defining the framework, and \individual businesses create the master data. It works best for large or medium organizations, and it requires agility in the creation of master data.
Velandia says that regardless of the approach, any organization should have robust data governance programs focused on critical data elements to derive long-term value.
“Data governance is not a one-time project, it’s a cultural skill,” he adds. “It needs to be built into an organization's culture and technical backbone, empowering business leaders and educating end-users to be effective data owners. The culture starts with the executives. Data governance is everyone's job, and this should be every company's motto. It is not the job of a bunch of data stewards or data governance managers. If you generate data, if you consume data, if you own data, you need to participate in protecting the data for the organization.”
Velandia explains that the idea is to focus not only on data quality as the main driver for the implementation of data governance programs, but to improve acquisition management and dissemination of data for key data domains.
“Some of the organizations I worked for used and implemented data quality management processes, starting from things like data profiles, to explore the data, to gain an in-depth understanding of it and to identify its issues such as inaccuracy, incompleteness, inconsistency, etc. Also, to define matrices related to establishing quantity, to understand the severity of those data issues. We have things like data matching to duplicate that basis, finding matching entities across several data sources,” he adds.
Velandia then divides the process for determining data quality into four key areas.
First, understand data quality issues using a data quality checklist. Second, identify the data source and systems where data is ingested, stored, and consumed. Third, identify data domains — like members, customers, or providers — that the data points and issues are related to. And finally, track, monitor, and quantify specific metrics to get a picture of the impact on the businesses.
Although data availability has increased over the years, sufficient attention has not been given to data quality.
And poor data quality can slow critical decision making, reduce operational productivity, and result in revenue loss, Velandia concludes.