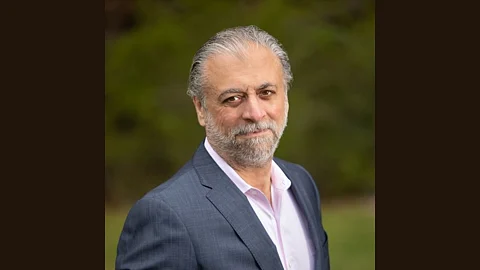
The top three things critical to a business's ability to compete in the market today are a holistic enterprise-wide data strategy, an architecture that helps enable broad access to effective, high-quality data, and a means for maintaining access and governance. And yet, for many organizations, creating this strategy and putting it into place remains an urgent challenge facing Chief Data Officers in multiple industries around the world.
There are many reasons why implementing such a strategy is challenging. New research from IBM found that one-fifth of IT leaders are not confident they have the right tools to locate and use data across their businesses, and increasing data complexity remains a challenge for 24% of organizations looking to invest in advanced technologies like AI. Organizations with a holistic data strategy are more likely to be innovative, competitive, and resilient thanks to advances that allow them to automate business processes, make better predictions, and empower teams to make smarter, more data-driven decisions.
For organizations without a strategy, putting data to work across an organization that fully realizes its potential will be very difficult. That’s why we at IBM worked to create a replicable, six-step framework for designing and implementing a data strategy. Based on insights from industry data leaders, this framework can help Chief Data Officers and their teams take advantage of best-in-class technologies while making the most of their efforts, talents, and strengths as an organization.
1. Understand your business objectives
As with any critical business strategy, having the buy-in, alignment, adoption, and execution from colleagues across your organization matters. That’s why meeting with C-suite executives and business stakeholders is your first step in helping your organization reach its objectives quickly and effectively. To help leadership see the strategic merits of data, clarify priorities as a collaborative, data-driven environment begins to take shape. Above all, be realistic: Too many organizations view digitization as a process they can complete in a few short months. Understanding your company’s current tech culture and strategies (and sub- and sub-sub-strategies, too) can help you plot a definitive and achievable course of action on data, AI, and applications.
2. Assess your current state
Organizations also need to take the time to identify all their shortfalls and vulnerabilities proactively. What is not working in the way your organization is currently working, and what is working? What are the barriers to delivering employees, leaders, and customers an authentic data-first experience? To surface and detect pain points in a data framework, design-thinking approaches can help your organization identify and bring strategic value to multiple use cases and across multiple lines of business or different teams. When I rejoined IBM in 2016, our business strategy centered on hybrid cloud and AI, which meant I needed to re-align and evolve our data strategy in light of our new focus. Design thinking forced us to confront critical questions, such as “How can we grow revenue in AI when many leaders don’t fully understand what an AI enterprise is or looks like?”
3. Map out data strategy framework
Your organization should break down the blueprint or map of its data implementation strategy into three segments. Begin with a target blueprint outlining how your organization will architect the technology solution. Secondly, create a target operating model that outlines how the new solution will work operationally; in other words, how it will be governed, maintained, and improved. Finally, develop an implementation roadmap that explains how your organization gets to its target blueprint and operating model in a step-by-step process. To further ensure buy-in from the rest of the organization, CDOs should be specific about where application modernization, automation, and AI can take the organization’s strategy to the next level.
4. Establish controls, empower data stewards
Robust governance and privacy capabilities help businesses thrive in a world where data can be overwhelming and pressures from consumers and regulators continue to grow. At its core, a data governance policy shapes protocols around data quality, privacy, and security and it allows organizations to show how AI is streamlining efforts to comply with regulations. Policy enforcement should be standardized so that everyone in the enterprise can speak the same language, regardless of business use, whether that data centers on clients, products, the workforce, or financials. It’s also critical to determine who within the organization will be the strongest allies and cheerleaders for smart data governance and strategy. The proper data stewards can help organizations work toward ensuring their strategy’s long-term success. Data governance is key to advancing the data strategy and driving data transformation forward. At IBM, we set up a Data Officer Council and have Business Unit Data Officers as partners to the Global Chief Data Officer. They ensure that IBM’s data strategy reflects Business Unit priorities and drive its adoption within the Business Unit.
5. Create integrated solutions
Development operations professionals typically work best in “short sprint” cycles with actionable milestones that help team members learn and adapt as they go. One approach is to push for smarter business outcomes with this simple, repeatable three-part process: Plan for one to two weeks with discovery workshops and data modernization; Prove over six weeks with a customer-driven use case set with actionable and learnable milestones; Adopt and scale with a test product tracked across internal stakeholders to ensure conversion. Minimum viable products give your team quick wins while working to move beyond siloed teams and thinking.
6. Scale your processes
The final step in successfully implementing a data-driven strategy is to scale your processes and tout your successes. This involves being confident about sharing wins and positive benchmarks while also being vigilant about re-skilling and hiring to ensure your strategy remains up to date over a long period of implementation. This work is ongoing and involves investing heavily in your existing talent pool. By 2026, for example, 60% of G2000 enterprises will have data literacy programs, including training to help employees spot misinformation and communicate or influence with data, to elevate their data culture.
A holistic, long-term data strategy is not something an organization can implement overnight. It requires significant buy-in from stakeholders across the organization, an honest assessment of its shortcomings, and a detailed blueprint for how it will advance toward its objectives. Once in place, the strategy must also be able to continue evolving in the face of new headwinds, governance challenges, or changes in the market, such as laws, regulations, and new technologies. Continuing to empower teams with new skills — and being quick to celebrate their successes and wins — can help provide the momentum your organization needs to implement a strategy and power it for years to come.
About the Author
Inderpal Bhandari is the Global Chief Data Officer at IBM. He has leveraged his extensive experience to lead the company’s data strategy to ensure that IBM remains the number one AI and hybrid cloud provider for the enterprise. Under his leadership, the Cognitive Enterprise Blueprint — a roadmap for IBM’s clients on their transformation journeys — was created.
Bhandari is an expert in transforming data into business value and improved customer experiences by delivering strategic, innovative capabilities that use analytic insights to enable growth and productivity. In 2017, he was named U.S. Chief Data Officer of the Year by the CDO Club, and he has been featured as an industry expert by The Wall Street Journal, The Washington Post, US News & World Report, CNN, and FOX.
Bhandari earned his Master of Science degree in electrical and computer engineering from the University of Massachusetts and holds a Ph.D. in electrical and computer engineering from Carnegie Mellon University.