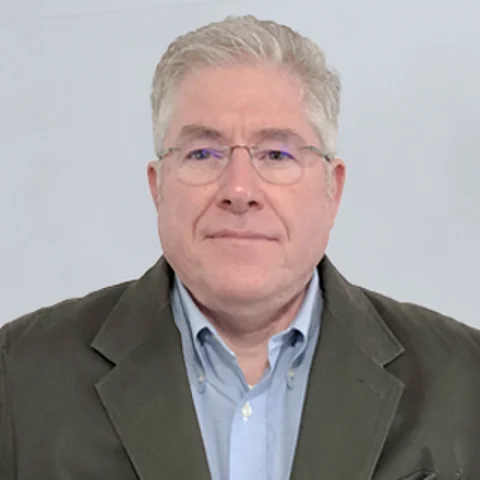
Summary: Speed is the top reason that I hear for scaling AI, but a direct approach to generating it often has the unintended consequence of reducing both productivity and business value. To scale AI across many business units and functions, companies need to balance three organizational behaviors that can conflict: speed, agility and control. In this article I cover the risks of these behaviors, the technology features that enable them, and the organizational structures that balance them.
When I talk to executives about why they want to scale AI in their companies, the overwhelming response I hear is speed. They want to go faster in product development, finance, marketing, supply chain, HR and every part of their business. Here are recent quotes from shareholder letters by a few top CEOs:
“The bigger benefit [of the cloud] is increased speed.” Jeff Bezos, Amazon.com
“We are training our people in machine learning – there simply is no speed fast enough.” Jamie Dimon, JPMorgan Chase
“The speed of change we see today is... faster than ever.” James Quincey, Coca-Cola
“Speed is how we deliver.” Kasper Rorsted, Adidas
“Greatly speed up our new focus on software and data.” Herbert Diess, Volkswagen
“Dramatically improve the speed with which we move new drug candidates forward.” Robert Bradway, Amgen
Too often companies new to AI take a direct approach to generating speed by hiring a few expert data scientists, providing them with public cloud accounts, and letting them loose to build custom solutions. We saw this recently at two Fortune 5 companies and while that approach worked for Google and Facebook, it fails for most businesses.
Risks of speed alone
- Time to ROI is too long and projects get canceled
- The data science experts are too detached from the business and don’t solve real, actionable problems
Risks of excessive control
Data silos and data duplication proliferate even further, as data that is valuable when joined gets separated
Shadow IT and AI teams emerge. This is sometimes called the Jurassic Park effect. In the movie Jurassic Park, restrictions were put in place to prevent proliferation but nature found a way around them. Similarly when IT or AI governance prevents business units from getting their job done, the business often finds a way around them.
Time to ROI increases as innovation is stifled
Data scientists perceive excessive access controls as politics, which is a top reason they switch jobs, so attrition increases.
Speed | Agility | Control |
|
|
|
Balancing speed, agility and control
Organizational structure helps keep these behaviors in the desired mixture by allowing managers to quickly move resources. The organizational structures for AI development that we see most often are illustrated here. Rectangles represent business units, business functions, a center of excellence or a center for enablement. The colored bars show the proportion of AI work and arcs depict collaboration between groups. (See image 2)
Distributed: little or no collaboration between business units or business functions
Silo of Excellence: deep AI expertise in one business unit or function, usually marketing or finance
Center of Excellence: a central, shared team that does AI work for all business units and functions
Center for Enablement (a.k.a. hub-and-spoke federation): a central, shared team that handles administrative tasks but doesn’t do AI projects for the business. Such tasks include infrastructure provisioning and monitoring, software licensing, regulatory compliance, best practices development, training, reusable template development, gamification of collaboration, process improvement, and maintaining a sustainable learning community.
Team of teams (a.k.a. mesh federation): peer-to-peer sharing between business units and business functions where each is responsible for their own connections and there is no central team
Companies with a high risk tolerance often begin with a distributed structure to explore many options concurrently while low-risk organizations start their AI journey with a silo or center of excellence. A silo usually has better initial ROI than a center simply because it’s closer to the business.[8] Tom Davenport, the author of many bestselling business books on AI, often recommends an AI silo of excellence as a first step. Companies that have scaled AI widely are three times more likely to use a center for enablement than those who haven’t scaled[9], and 90% use interdisciplinary teams on AI projects.[10] Team of teams might someday become common in large companies in competitive industries since it has the best aspects of each structure and promises to “combine the agility, adaptability, and cohesion of a small team with the power and resources of a giant organization.”[11] So far though, it's the least common organizational structure we see in practice.
In conclusion, most businesses want speed, agility and control. AI strategists should determine the mix that’s best for them in order to broadly scale AI.
About the Author:
Doug Bryan (Doug.Bryan@Dataiku.com) recently joined Dataiku as an AI Strategist to help companies navigate the path from 100 to 1,000 AI practitioners. His background includes lecturer in computer science at Stanford University, leader of the product recommendations team at Amazon.com that generated $2B in incremental revenue per year, Accenture Labs, Vice President of Analytics at Hearst Communications, and Senior Vice President for Data Science Products at Dentsu International. He has 25 years of data science experience and has done consulting worldwide in many industries for clients such as Barclays, Nationwide Insurance, Marks & Spencer, L.L.Bean, PNC Bank, Mazda, Wells Fargo, Overstock.com, Motorola, Experian, Discover Financial Services, Rogers Wireless and Carnival Cruises.