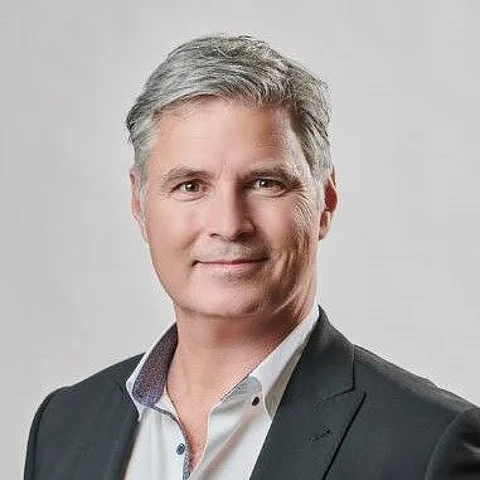
(US and Canada) Mario Cantin, Founder and Chief Data Strategist at Prodago, speaks with Robert Lutton, Sandhill Consultants Vice President, about different approaches to data governance, why data and analytics are tied together, how Prodago implements analytics to address challenges, and how lean data and analytics governance operating models help organizations.
Data governance is about setting policies and enforcing them, says Cantin. Numerous organizations struggle as they think value comes from implementing a data governance structure like stewardship organization, business glossary tool, or data quality management, Cantin adds, while he thinks that is a misconception.
The ultimate value, he believes, comes from the trust, collaboration, and agility of the organization to use data. He mentions that Prodago stands for project data management, and it ensures that the project gets value from data governance.
Next, Cantin states that data governance initially meant governing the attributes of data, comprising data lineage, stewardship organization, metadata management, and data quality issue management. He adds that the last decade saw the enlargement of data governance to disciplines like ethics, privacy, and security, which are different facets of data management that require governance.
Cantin points out that the work is more about governing data management than governing data. This is because data management includes everything from metadata management to security and ethics, he says. Governing data management and the specificities of analytics goes beyond quality, privacy, and ethics, Cantin stresses, saying that organizations need to govern bias management and human agency as well. That way, data and analytics governance go together to ensure that all essential facets of data management are covered, he continues.
Emphasizing analytics, Cantin indicates that Prodago supports organizations in their advanced analytics and AI projects. He asserts that it is a lean governance framework that includes enterprise and project governance. Without governance, 80% of the AI initiatives fail to develop due to the misalignment of the developing model with business objectives, he adds.
Cantin elaborates that Prodago helps organizations plan, execute and build oversight about their AI and advanced analytic projects. The platform not only plans metadata management, data quality, and privacy aspects, but also defines activities of human agency, bias management, and model management, he says.
Next, Cantin mentions that the lean data and analytics governance model is critical in helping organizations implement best practices while designing solutions. Being lean ensures implementing the minimum governance required to succeed in the AI initiative, he says.
Finally, Cantin highlights that injecting too much governance can also kill the initiative. He urges that the governance level be adjusted based on the essential laws and organizational maturity to build it as organizational capabilities grow.