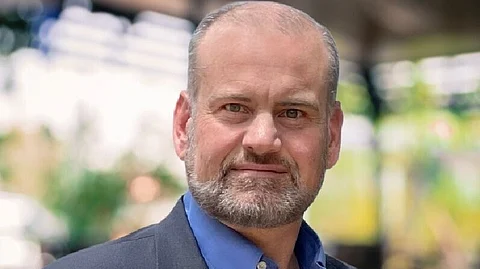
In a recent study by Eloundou et al, researchers forecasted the impact that Large Language Models (LLMs) like ChatGPT might have on the labor market. This is a critical question over the coming decade, as Artificial Intelligence (AI) appears to finally be moving out of the lab and into prime time. The study noted the extremely impressive performance that ChatGPT has shown in passing a wide range of professional examinations which are typically used as barriers to entry in a majority of knowledge worker careers. Such performance naturally leads to the question:
Will ChatGPT lead to major disruptions in the market for human labor, and in particular, will this technology dislocate many of our society’s highest-paid workers?
The study compared the abilities inherent in ChatGPT to the assessment of tasks and skills required in over one thousand occupations. It reasoned that those occupations which could most easily be performed by ChatGPT were the most at risk of being replaced. The findings of the report are disheartening, if not downright dire.
It suggests that there is a wide range of high-paying professions that are likely to be supplanted by ChatGPT, if not rendered completely obsolete. Ironically, business writers are among those being rounded up for orderly disposal by the ChatGPT Skynet.
This study leads to several nagging questions:
Will great swaths of highly educated, middle-class taxpayers suddenly find themselves unemployed, and unemployable, due to these new omniscient and omnipresent digital workers?
Everything old is new again
While the research approach and source material are sound, they do not necessarily reflect the realities of how businesses consume the inputs of production (labor, capital, raw materials, knowledge, and organization) to create business value.
Anyone who has worked in process engineering, reengineering, process improvement, lean, Kaizen, six sigma, digital transformation, or every other business trend since World War II can attest to the observation that every new information technology faces a significant headwind in realizing its full potential.
The following are six considerations to bear in mind as you weigh the use of ChatGPT for knowledge worker automation:
1. Excelification
Modeling and automating organizations and processes are nothing new, they made Henry Ford fabulously wealthy over one hundred years ago. Yet, for all of our modeling ability, many organizations still struggle to effectively integrate new technologies and automate their operations. If we have the data and the tools, why do we still fall short?
The American writer Mark Twain, famous for both his wit and his pragmatism once wrote, “There are three kinds of lies: lies, damned lies, and statistics.” If Twain was born in the 20th century, earned his MBA, and spent a few decades creating financial and operational models in Excel, I can say with certainty that he would add “Excel” as his fourth category of lies, and he would rank it as even worse than statistics.
No matter how good we may be at attempting to model our organizations, and in particular, the impact that any innovation might have on our operations, one variable always seems to ruin our models – Us.
Individually or in groups, humans have a predictable unpredictability that often foils our best-laid plans. Generally, the things that ruin these plans are not those reflected in the rows and columns of our Excel models; if they were, then we made bad models. Instead, those items that tend to muck up the works are those that we miss in our models, consciously or not.
We should expect to find similar issues in nearly every attempt to model business automation, particularly whenever statistics and Excel are involved. Hence, when people attempt to model how technology will impact human organizations, expect that the variables that make us most human will be those that most mess up our equations.
2. Collapsing means mean collapsing means
As statistical engines, LLMs tend to generate results that are the average (or mean) that they learned from the data that they accessed. Over time and with use, they can potentially generate better than mean results, but only if their ongoing development is carefully managed. As I have covered elsewhere, this is generally not the case with Generative Artificial Intelligence (AGI) tools such as ChatGPT.
Such LLMs determine a response’s “correctness” based on its popularity (as with search results) rather than its actual “rightness.” This is like judging the correctness of a scientific theorem based on its score on Rotten Tomatoes!
In either case, statistical engines typically collapse to the mean in their responses. Over time, they eventually have a single most average response to a question, regardless of the variations in how it is asked. Unless this average or mean response is purposefully and artificially driven to a better answer, the average answer will generally be the result. In an age where organizations are specifically seeking diversity of thought, LLMs inherently provide the exact opposite.
The implication of this is that organizations that use LLMs without oversight will find these tools to rapidly lose their value. A team with a dozen different employees might produce a wide range of responses to a question posed by a customer – some average, some worse, and some better. Good organizations seek out and use the best answer provided by a variety of employees. This means of generating a better-than-average (better than mean) result may be lost by organizations that embrace LLMs broadly.
They will generate very consistent yet very average results and so will all of their competitors. As everyone adopts them, LLMs will level the playing field in all areas of competition dramatically. In such a marketplace, only those who maintain these systems with the highest levels of knowledge and oversight will consistently generate better results.
Meaning, your means of improvement (i.e., people) will be far more important to you, rather than less. Your people will become far more important to your competitiveness precisely because you replaced them with a technology that gave the appearance of being superior. On average, ChatGPT might be superior to average people. But when it is not superior, it will be shockingly worse.
3. Knowledge work is lumpy
The research looked at those tasks normally performed by knowledge workers. ChatGPT is particularly good at performing tasks such as looking up data, creating documents, or answering queries. Hence, it could likely replace workers who perform these tasks, such as auditors, writers, or customer service representatives.
The research compared those tasks for which LLMs excel and compared them to the tasks that were typically associated with certain roles. The resulting overlap suggests those areas where organizations could replace humans with LLMs most effectively. However, this approach misses a lot of important details.
After centuries of optimization, manufacturing lines move forward at a constant pace, with inputs designed to be added at a predefined rate. Unlike manufacturing, knowledge work tends to occur in fits and starts. Organizations have attempted to automate knowledge work for half a century. What remains, tends to not follow a metronome of business value creation. In short, knowledge work is lumpy.
This inconsistency is what frustrated so many efforts at Robotic Process Automation (RPA) over the last decade. One purchase order might require mere minutes of review, while another may require weeks. The nearly endless variations in the content and context of knowledge work mean that it cannot have a steady pace. Without a steady pace, an improvement in an upstream task might do little if anything to improve a downstream task. Often an improvement in one step simply led to a longer wait further down the line.
In the early days of RPA, there were a small number of knowledge worker tasks that required a large number of people to perform the same task in parallel. In these instances, RPA shined. But these tasks were few and far between, and automating them simply caused backups elsewhere in the process. RPA tended to emphasize and enhance the lumpiness of knowledge worker processes. ChatGPT will almost certainly do so with even greater effect.
3. Knowledge workers are lumpy too
Just as knowledge tasks are highly variable, so are knowledge workers. We all know situations when a certain customer calls or a particular problem arises, that we want it to be handled by a specific person. We can try to make knowledge work and knowledge workers fungible but we also know this is an illusion.
Certain people have unique skills, experiences, and knowledge that simply cannot be replicated by a statistical engine like ChatGPT. LLMs like ChatGPT flatten out and nullify such unique inputs, rendering them unusable in precise situations when a unique approach will make the difference between success and failure. Like RPA, LLMs can replicate a knowledge worker’s, “what to do,” but they cannot replicate the “why to do it.” Without both, ChatGPT will fail to make the right judgment call precisely on those occasions when it is most important for it to be right.
4. Fast, cheap, or good
Engineers from NASA used to have a saying: Fast, Cheap, Good, pick any two. They recognized that in complex systems there is always a balance between what is desired and what is possible. The greater the complexity of a solution and its inputs, the more difficult these decisions necessarily become.
Information technologies often promise “better, faster, cheaper,” and often they do deliver on at most two of these promises. Rarely can they deliver on all three. Enterprise Resource Planning (ERP) was pretty good at automation but failed at being fast or cheap. Cloud computing is fast and good, but definitely isn’t cheap, except for specific and optimal workloads.
Often technologies appear to provide all three benefits at the same time, but equally often we are being deluded by our Excelification. Our models are missing one or more critical variables, and we don’t question them because we like the story that they are telling us. But, any time we are told a new technology is simultaneously “fast, cheap, and good,” we should be very skeptical in the absence of a lot of evidence.
One of the things that is rarely modeled in such analyses is the cost of fixing things if something goes wrong. The less we prepare for such events, the more likely they are to occur, and the worse the results when they do. Relying on an LLM in such instances leads us back to the same paradox of average results.
If ChatGPT gave us an average answer, and that answer proved to be wrong, how can ChatGPT help get you out of the mess that its prior response created? As Albert Einstein famously stated, “We cannot solve our problems with the same thinking we used when we created them.” If Einstein couldn’t do it, neither can ChatGPT. And if you replaced your workers with ChatGPT, who then will come up with the new thinking required to fix the problems ChatGPT created?
5. If I could be like Mike
Growing up in the 1980s and 90s, Michael Jordan was the first real sports superstar that I had known. A commercial famously shared how most kids wished, “If I could be like Mike,” what would I do? Thirty years later, that ‘Mike’ that children may wish to grow up and emulate may not be a basketball player, but instead may be a world-famous data scientist or software engineer.
A decade ago I wrote an article discussing the future of knowledge worker labor in a world with widespread use of AI. I described the ‘baller effect,’ where in hyper-competitive fields all demand and hence all income is concentrated in the very smallest and top percentage of workers, to the exclusion of everyone else.
We readily see this in professional sports, where top ‘ballers’ earn tens of millions per year, and the people below them earn little to nothing. The same market effect also exists for actors, singers, and other high-profile professions, and now is coming to the world of knowledge workers.
If your protection from errors made by ChatGPT necessarily requires someone more knowledgeable and skillful than ChatGPT, how do you ensure that you have such people on your side?
Certainly, ChatGPT can readily and effectively replace your below-average employees, but it can and will equally weigh down any of your remaining above-average employees. Using a statistical engine like ChatGPT becomes a Faustian bargain that will either deeply commoditize what is currently value-added work, or force you into an intellectual arms race that will grow ever more expensive.
ChatGPT can rapidly create 80% solutions to your needs. If that is good enough, you should take it to market as-is and take advantage of the speed and cost provided. This not only allows you to eliminate these workers, it necessitates that you do. However, this will rapidly commoditize this output, and you will have to innovate elsewhere, with higher-order thinking.
Conversely, if you use this ‘80% solution’ as an input into your very best human workers you will be able to create even greater products and services, but you will be even more dependent upon these rare, and more differentiated resources to maintain a competitive edge. Again, this will be a talent and skill arms race facilitated by ChatGPT but not winnable by ChatGPT alone.
As stated above, LLMs like ChatGPT provide enormous leverage against human talent. It simultaneously allows us to mostly replace large numbers of people, and significantly enhance the outputs of our very best people. The logical conclusion of both of these effects is that employers will be highly motivated to hire and retain the very best knowledge workers, regardless of cost, to the exclusion of anyone less skilled.
If ChatGPT can multiply the outputs of your best people by a factor of a thousand, then someone twice as good as average isn’t twice as good, they are two thousand times as good.
6. Get busy livin’ or get busy dyin’
As the character Andy Dufresne famously quoted from prison in Steven King’s movie Shawshank Redemption, when faced with a nearly impossible choice the choice was actually easy — “Get busy living or get busy dying.” For organizations, the use of ChatGPT is optional just like using the Internet, e-mail, social media, or RPA. You can choose to not engage, but this will emphatically represent the “get busy dying” approach to the problem.
In the movie, Dufresne applied time, knowledge, experience, ambition, attention, and focus to a plan of escape that ultimately collected for him a highly improbable outcome – freedom from prison. The same is true of dealing with any complex and challenging problem. This mix of ingredients is eminently human, and is unlikely to be replicated in any machine or artificial intelligence any time soon, if only because we do not know where they come from in our own minds.
Success with these tools will require that we embrace them without becoming wholly dependent on them. Will they have a significant impact on the knowledge worker labor market? Invariably, yes. Will it be the catastrophe that some are now predicting? Maybe.
Technology always displaces labor in the equation of value production. The only difference between ChatGPT and the spinning wheel is the speed that we, the next generation of Luddites, have to prepare ourselves.
About the Author
Christopher Surdak is an award-winning, internationally recognized transformation expert. He leverages leading-edge technologies to drive results-oriented digital transformation for organizations of all sizes, industries, and regions. Most recently, he served as the Acting Chief Technology Officer with The White House Office of Science and Technology Policy.
Surdak is the author of the books “The Care and Feeding of BOTS," “Jerk: The Digital Transformation Cookbook,” and “Data Crush: How the Information Tidal Wave Is Driving New Business Opportunities.”
He holds a Juris Doctor from Taft University, an Executive Masters In Technology Management and a Moore Fellowship from the Wharton School of Business at the University of Pennsylvania, a Master’s Certificate in Information Security from Villanova University, and a Bachelor of Science in Mechanical Engineering from Pennsylvania State University.