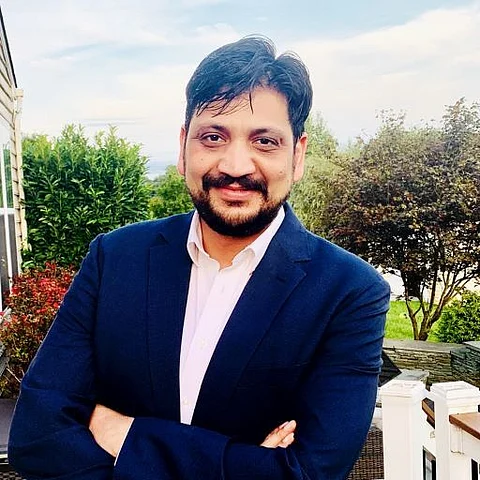
When Amit Garg and Neel Butala met years ago at the Yale Entrepreneur Institute as students, they realized big data’s potential for improving the healthcare sector. But they were also faced with a problem — relevant data was expensive and difficult to access. Their brainchild, HiLabs, was born as a solution to this key problem in 2014.
Garg, co-founder and CEO of HiLabs, is a technologist with deep experience in health plan operations. He has a professional passion for simplifying data mining in healthcare and generating critical insights from unorganized healthcare data. Butala is an MD/MBA with a background in economics. He shares Garg’s passion for finding ways to use cutting-edge data science and machine learning (ML) to improve healthcare outcomes.
For HiLabs, the initial challenge was discovering the best ways to apply AI and ML to add value in a healthcare setting. “Our platform and technology have always been best in class. However, without a deep understanding of business context and a customer that is willing to learn with you, it is difficult to find the area in which you can add the most value,” Garg says.
The team soon realized that most clinical, claims, and provider data were too inaccurate or incomplete to perform high-quality analysis. Backed by the combination of the clinical expertise and technological perspective of the co-founders, HiLabs pivoted to solving the health data challenge using the same technologies and techniques.
Garg says that the journey so far has been very exciting. “Along the way we have learned from our experience to constantly iterate our approaches to get more accurate data and add more value to our customers. We have been successful in significantly improving several areas of health plan operations, including improving member-facing provider directories, improving the accuracy of claims payments, and onboarding and analyzing vast volumes of clinical data,” he notes.
Today, HiLabs stands at the intersection of healthcare and data, which sets them apart from the competition. “Healthcare and data intersect everywhere at HiLabs,” Butala says. “We are deeply focused on the healthcare industry and believe that is what gives us an advantage over more generalist approaches and companies.”
HiLabs primarily works with health plans. The engagement can be better described as collaboration with a highly iterative approach. “We have the most success when we have strong input from our health plan teams,” Butala adds.
Key problems solved by HiLabs for healthcare clients:
Ensuring members of health plans get connected with physicians without friction.
Reducing surprise billing.
Reducing administrative burden on health plans and providers in the provider data quality area.
Ensuring health plans pay claims accurately to their provider partners.
Discovering data or care gaps that health plans and providers can close with minimal effort.
Improving the accuracy of value-based care payments to providers in VBC arrangements.
Optimizing outreach to patients to drive better adoption of preventative care.
Analyzing the cost of progressive diseases and how interventions may both improve health and lower costs over the longer term.
HiLabs’ client onboarding journey begins by explaining the technology and the general approach of applying AI/ML to the healthcare space. Next, the team works with the customer to pick an area from HiLabs’ library of successful use cases to implement.
“We work with the customer, engaging in a four-to-six-week Proof of Concept so that they can see the results our solutions can produce in their own context. All POCs include results from real customer data and we strive to have several iterations in the POC so that when production begins, we are already producing high-value results,” Garg says.
The POC is followed by a production agreement that includes the usage of HiLabs’ core platform, and all support and operations are included.
While the benefits from higher quality data for HiLabs' clients cannot be overstated, there are certain key tangible benefits. Some of these include lower compliance fines and audit failures, lower operational costs from increased automation, improved analytics and insights generation, and more satisfied members and providers.
Sharing his view on the way forward and how the landscape could change in the coming years, Garg adds, “We believe that, as our solutions improve data quality, we will soon cross the threshold where our original vision can be realized. We can soon use high-quality upstream data inputs to truly drive lower cost, higher quality healthcare for everyone.”