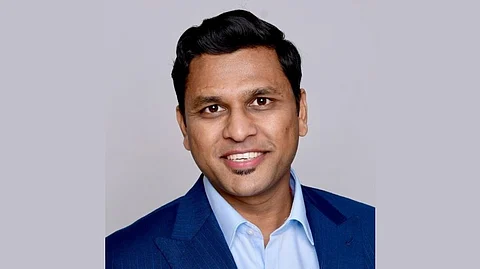
In today's fast-paced business landscape, where data reigns supreme, the role of data and analytics teams has evolved from being mere support functions to becoming pivotal players in organizational success. Enterprises recognize these teams' value in harnessing data for informed decision-making and competitive advantage.
Organizations are transitioning themselves into being product-centric vs. traditional project-centric approaches to achieve value in a consistent and scalable manner.
However, the journey from a project-centric to a product-centric mindset has hurdles. The organization's goals and needs frequently determine whether data analytics use cases are project-centric or product-centric. Companies usually combine these tactics based on their need and organizational fit.
This article will dissect organizations' most common challenges during this transformation and present proven best practices to steer your teams toward success.
Transitioning from a project-centric to a product-centric model entails a cultural shift that touches every aspect of an organization. It goes beyond just tools and technologies and requires changing mindsets, processes, and behaviors.
The goal is to treat data and analytics capabilities as strategic assets that continuously deliver value and have a defined life cycle rather than one-off projects with defined endpoints.
Let us go through some common challenges organizations see and the best practices to overcome those.
Challenge: Resistance to change is perhaps the most formidable obstacle. Teams accustomed to project-based thinking may find it challenging to adapt to the continuous delivery model.
According to my understanding of agile methodologies, agile is incremental and iterative, so a continuous delivery model should not be challenging. However, there might be a challenge meeting the deadlines as the entire idea of agile is to have faster delivery times, which when prodded into, usually leads to miscommunications across multiple teams as each team works at its own pace. I have highlighted the solution below.
Companies already running agile projects have a better alignment on agile expectations, but the definition of a data and analytics product is still very loose and broad across organizations.
Best Practice: Foster a culture of collaboration, transparency, and accountability. Encourage cross-functional teams to work together towards common objectives, fostering a sense of ownership. The first step will be to define the various forms of Data and Analytics products, which will be the responsibility of the Data and Analytics Leader. Then, establish standards and a framework for ideating, developing, deploying, scaling, and declining Data and Analytics products.
Everyone within the Data and Analytics organization must lead the change toward a product-centric culture by overseeing product strategy. The task could potentially be in partnership with product managers and/or corporate learning and development teams.
Challenge: As organizations mature their journey into building data and analytics products, the definition of a data product or an analytics product could be clearer. Lack of data and analytics product lifecycle will drive operational costs.
Best Practice: Standardizing the definition of the “Data Product” or “Analytics Product” can help in multiple ways. One of them will be that Data and Analytics products can be separated from any other product forms the organization may be invested in. In addition, a defined life cycle can help avoid unnecessary maintenance of assets deployed once and never looked at.
Challenge: Without clear ownership, ensuring accountability and driving results is challenging. In a project-centric model, ownership shifts from one team to another (development to deployment, to operational maintenance) after completion.
Best Practice: Appoint product owners in partnership with key business leaders and stakeholders with a holistic view of the data product's lifecycle. They should be responsible for its development, maintenance, and performance, ensuring alignment with business goals.
Appointing individuals with an in-depth understanding of the product's function and consumer requirements can help achieve a holistic view and complete ownership.
Challenge: Weak data governance can lead to inconsistencies, poor quality, and compliance issues, hindering the transition to a product-centric approach.
Best Practice: Establish robust data governance frameworks encompassing data quality, lineage, privacy, and compliance. Provide training and resources to ensure adherence across the organization.
Challenge: Traditional project-centric models often lack mechanisms for continuous feedback, making it difficult to adapt to changing business needs. The project-centric feedback collection may not usually match the product-centric goals throughout.
Best Practice: Implement Scrum, Kanban, or other agile methodologies that may combine these two to facilitate iterative development and frequent feedback loops. Encourage regular communication with stakeholders to ensure alignment with evolving requirements.
The Product Owner’s vision and strategy define the product life cycle, which can guide the frequency of collecting feedback and using it for relevant future features or product versioning.
Challenge: Balancing resources between ongoing product maintenance and new initiatives can be complex.
Best Practice: Implement resource allocation models considering strategic initiatives and operational maintenance. Prioritize based on business impact and allocate resources accordingly. Prioritization becomes key in this step. Identifying high-impact, high-value features that drive positive customer impact and delivering them must be the approach.
In Data and Analytics products, it usually comes down to delivering the products that support high-impact business stakeholders like the executive leadership or enhancing a dashboard or a data pipeline that supports a new marketing campaign.
Challenge: Shifting from project-based Return on Investment (ROI) metrics to long-term product success metrics requires a shift in mindset.
Best Practice: The measurement of success and return on investment (ROI) assumes great significance in the move from project-centric to product-centric models, as it offers concrete evidence of the efficacy of this transformation.
Define clear Key Performance Indicators (KPIs) that measure data products' sustained value and impact. Focus on metrics such as user engagement, data quality scores, and business impact over time. Create and implement product-centric key performance indicators (KPIs), milestones, and other success metrics that can be tracked through the product life cycle.
These may include customer satisfaction, product adoption, utilization, customer retention rates, and customer lifetime value to name a few.
Transitioning from a project-centric to a product-centric organization in data and analytics is a substantial undertaking. However, by recognizing and addressing these common challenges head-on, organizations can pave the way for a more agile, value-driven, and competitive future.
Having a clear definition of the product, embracing a culture of collaboration, investing in robust data governance, training business and data and analytics teams to think in terms of the holistic product lifecycle, and adopting agile methodologies are just a few of the proven best practices that propel your organization toward success in this transformative journey.
Remember, the keys to success lies in the tools you use and the mindset with which you wield them.
About the Author:
Phanii Pydimarri, Head of Strategic Planning and Partnerships at Health Care Service Corporation (HCSC), is a Global Digital, Product, and Data Analytics Leader with successful experience driving Digital Transformation, product commercialization, growth strategies, and end-to-end Data Management with key focus areas such as Strategy, Data Analytics, Business Transformation, Product Management, and Customer Success.
Pydimarri started his career as a Data Analytics Consultant, traveling the U.S. and working for various industry clients. He strongly believes in economies of scale, is outcome-driven, and is passionate about solving critical business challenges using Digital and Data technologies. He has substantial experience using Data Science to improve critical operational challenges and has experience standing up Data Governance programs across various public and private sector organizations.