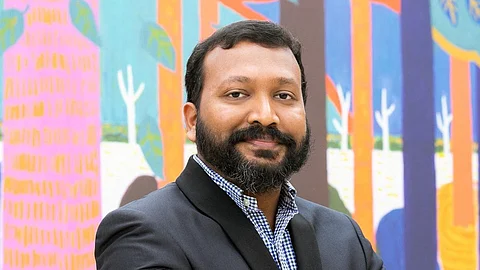
Karthikeyan Ilangovan is a data analytics leader with 15 years of experience in leading cross-functional teams in the retail, telecom, food, and financial services sectors. He currently leads Business Intelligence Modernization efforts at Neiman Marcus, an American luxury retailer headquartered in Dallas, Texas.
Ilangovan is passionate about data democratization, making data widely accessible, understandable, and actionable.
In conversation with CDO Magazine, he opens up about his approach to decommissioning legacy data and reporting systems, enriching data sets and pipelines, building guided analytics with modern dashboards, automating manual data processes, and driving business insights to increase revenue.
Read the full interview for insights on elevating bespoke analytics, the GenAI buzz in analytics and automation, challenges in data democratization and literacy, and how organizations can reach analytics maturity.
How do you define your role as a thought leader in the data analytics space, and what key principles guide your approach to solving complex business problems?
I am a thought leader who inspires innovation, fosters a culture of data-driven decision-making, and guides organizations in harnessing data while adhering to industry standards and best practices. Personally, the following principles have proven to be effective in solving most business problems.
Data-centricity: Recognizing that data is at the core of every decision, I prioritize its quality, relevance, and accessibility.
Holistic analysis and diversity: Looking at the problem from multiple perspectives and considering all relevant factors - not just focusing on symptoms but understanding the root causes. Also, engaging diverse perspectives and expertise, leading to more comprehensive and effective solutions.
Stakeholder engagement: Involving key stakeholders in the problem-solving process. Understanding their perspectives and needs ensures that the solution is well-rounded and addresses the concerns of all parties.
Agility and flexibility: Being adaptable and open to changing course if new information comes to light or if the chosen solution isn't providing the desired results.
How did you adapt your strategies to different industries, and what common challenges did you encounter?
Each industry presents unique challenges and opportunities but certain principles remain consistent. A key aspect is the centrality of data in decision-making across all sectors. Regardless of the industry, data quality and relevance are paramount.
In financial services, the focus often revolves around regulatory compliance, risk management, and optimizing financial processes. Telecom demands strategies for customer retention and network optimization. The food industry prioritizes supply chain efficiency and quality control, while retail emphasizes customer experience, marketing, and inventory management.
The common challenges include data silos, legacy systems, and the need for cultural change towards data-driven decision-making.
Building cross-functional teams and fostering collaboration are essential in every industry. Moreover, staying updated with industry trends and emerging technologies, and being able to adapt and innovate is a common thread that runs through all sectors.
You're passionate about data democratization. How do you define the concept, and what steps do you take to make data widely accessible and are there any unique challenges?
Data democratization is about making data widely accessible and usable across an organization, ensuring that employees, regardless of their technical background, can easily access and leverage data for decision-making. It's about breaking down data silos, promoting transparency, and empowering individuals to harness data's insights.
The following 7 steps worked well for me:
Implement unified data platforms, simplifying access by consolidating data sources and tools, and creating an industry-standard KPI framework.
Promote self-service analytics using user-friendly tools like Tableau or Qlik.
Prioritize training and education for enhanced data literacy.
Establish robust data governance for quality and security.
Ensure role-based access controls.
Implement data catalogs for efficient dataset discovery.
Foster feedback loops to continually enhance data accessibility and usability.
The challenges include resistance to change from employees accustomed to traditional data access models, ensuring data privacy and compliance, and addressing the scalability of self-service tools as the organization grows.
For organizations aiming to reach analytics maturity, what key pieces of advice would you offer in terms of strategy, technology adoption, and cultural change?
The path to analytics maturity remains consistent, but adaptation is key. Organizations need a holistic approach. Here are a few steps:
Begin with a clear data and analytics strategy aligned with your business objectives.
Prioritize robust data governance for quality and security.
Invest in modern and scalable technologies like cloud-based platforms and BI tools while nurturing a culture of continuous learning.
Democratize data access, ensuring it is available to all while implementing role-based controls.
Embrace change management to foster data-driven decision-making and collaboration.
Establish measurable KPIs, gain leadership support, and adhere to data ethics.
Remember that analytics maturity is an ongoing journey, requiring regular evaluation and improvement.
What’s an average day like in your work life?
A typical workday includes blending strategic vision, effective team management, and seamless collaboration across various departments. Days are usually peppered with meetings where I chart the strategic and tactical course for our analytics teams, ensuring our objectives remain tightly aligned with the goals of our organization. This includes monitoring team performance and deliverables, and guiding with direction to ensure we're consistently exceeding expectations.
I'm also engaged in project prioritization, hands-on data analysis (when required), managing budget and forecasting processes, exploring emerging technologies and external partnerships, management of analytics infrastructure, and building strategic alliances with key business leaders to integrate analytics into broader corporate strategies.
What were the key challenges facing Neiman Marcus's existing BI systems, and how are you approaching the modernization process?
Our BI systems were outdated technology suffering from scalability limitations, data fragmentation, and governance issues. The systems hindered agility and couldn't efficiently handle the growing data and reporting needs.
We began BI modernization by first upgrading existing BI platforms to incorporate the latest capabilities and security measures. We then worked on a plan to decommission legacy systems with fewer users and activity, which streamlined data and reporting with fewer systems and improved reliability.
The self-service analytics capabilities with Tableau empowered business users to create reports and visualizations, reducing core IT dependency. Qlik Cloud was introduced as the enterprise reporting system, serving as the single source of truth across the organization for both operations and executive reporting and leveraging code-free user experience and AutoML capabilities.
We also partnered with product teams to introduce a new data governance tool that simplified data discovery with a data catalog, enabled us to see the full story of data with data lineage, and solidified data governance pairing well with our Snowflake, Qlik, and Tableau ecosystems.
What are some practical applications of GenAI in analytics that you're excited about, and how do you envision it impacting Neiman Marcus's operations?
Neiman has a loyal affluent clientele where 40% of the sales comes from the top 2% of customers who spend about US$ 27,000 a year and 80% of top customers have at least US$ 1 million of net worth. Customer relationship and personalized customer experience are key drivers in revolutionizing the shopping experience.
GenAI can analyze vast amounts of customer data to create highly personalized shopping experiences. By understanding individual preferences and behaviors, we can offer tailored product recommendations, pricing, and promotions, leading to increased customer engagement, loyalty, and sales.
In retail, the technology can aid demand forecasting and inventory management ensuring that popular items by season are always in stock while minimizing overstocking and markdowns, ultimately improving profitability.
Merchandising and Assortment Planning would be another domain where GenAI can analyze customer preferences, fashion trends, and sales data to assist in merchandise selection and assortment planning. This ensures we offer the right products in the right season, locations, and right channels. GenAI can also optimize marketing campaigns by analyzing customer behavior and preferences maximizing their impact and ROI.
How can leaders prepare their teams and adapt their data infrastructure to leverage the potential of GenAI in data analysis?
Leaders should concentrate on strategic areas within a concise framework, maintaining a continuous feedback loop. These include:
Education and training: Equipping the team with knowledge about GenAI applications in data analysis and understanding the ethical implications.
Infrastructure adaptation: GenAI demands robust computational resources. Leaders should assess and upgrade their data infrastructure as necessary.
Data governance: Establish and enforce stringent data governance policies maintaining data accuracy, consistency, and security, laying the foundation for reliable and ethical AI use.
Collaboration and ethical practice: Encourage a culture of collaboration between business, data, and IT professionals. This approach fosters innovative applications of GenAI. Simultaneously, emphasize the ethical use of AI, addressing critical issues like data privacy and algorithmic bias.
Throughout your career, you've led successful cross-functional teams in various industries. What are your key strategies for fostering collaboration between data teams and other departments?
First and foremost, clear communication is key. Establishing regular meetings and shared digital platforms ensures open dialogue. It's vital that team members from all departments feel comfortable sharing ideas and concerns.
Aligning on shared goals has been another cornerstone - setting objectives that necessitate contributions from both data teams and other departments. Joint problem-solving sessions and offering cross-functional training have greatly enhanced mutual understanding, respect, and empathy. Additionally, acknowledging and celebrating collaborative achievements has proven essential in reinforcing teamwork.
Mention three things data leaders should keep an eye on in 2024.
Advancements in AI/ML: Stay informed about these advancements as they can significantly impact how data is analyzed and utilized. Keep up with trends in AI ethics, explainability, and the growing use of generative AI models.
Data privacy and regulation compliance: With increasing global attention to data privacy and security, data leaders must ensure compliance with evolving regulations like GDPR, CCPA, and others. The rise in consumer awareness about data rights will also drive changes in data collection and usage policies.
Emerging technologies and infrastructure: New technologies such as quantum computing and edge computing can offer novel ways to process and analyze data. Data leaders should understand how they might enhance or disrupt current data practices. Robust cloud infrastructures and efficient data storage solutions will also be areas to focus on.
Name a data leader you look up to, and why?
That would be Swedish physician, academic, and public speaker Hans Rosling. Rosling had a unique talent for bringing statistics to life, often challenging common misconceptions, and revealing the true story behind the numbers.
His book "Factfulness" and his work with Gapminder Foundation revolutionized the way we understand global trends and development issues. His ability to distill complex data into clear, vivid visualizations and narratives is a model I deeply admire and try to emulate in my work.