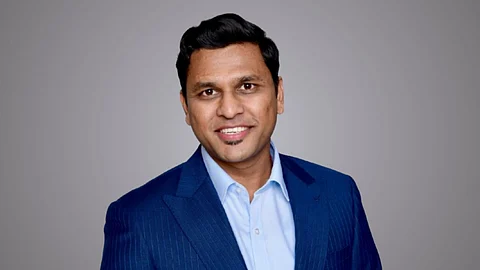
As AI continues to dominate boardroom discussions, the pressure to implement cutting-edge solutions and articulate a robust AI strategy has never been more significant. However, as C-suite executives and board members, it's crucial to recognize that the actual cost of AI extends far beyond the initial investment in technology.
While the focus often centers on the immediate expenses of developing and deploying AI models, the long-term costs of maintaining these models — and their environmental impact — are equally significant.
AI's carbon footprint, driven by the energy-intensive process of training and running machine learning models, poses a financial and ethical challenge. As stewards of your organization’s future, it’s imperative to consider how these unseen costs could affect your bottom line and corporate social responsibility.
The conversation about AI must expand to include sustainable practices that balance innovation with environmental stewardship, ensuring that your AI strategy is effective and responsible.
Training machine learning models, especially deep learning models, requires vast computational resources. These resources are not just expensive in terms of time and money, they also consume large amounts of energy. For instance, training a single large natural language processing (NLP) model can emit as much carbon dioxide as five cars over their lifetimes.
To put this into perspective, a study by the University of Massachusetts Amherst, found that training a single AI model can emit over 284 tons of CO2, equivalent to the emissions from 20 round-trip flights between New York and San Francisco.
This energy consumption is driven by the need for robust data centers that host the computational infrastructure. These data centers require cooling systems and continuous power supply, contributing to their carbon footprint. The energy demands increase with the model's complexity, the dataset's size, and the number of iterations required during the training process.
The carbon footprint of AI is not uniform; it varies depending on several factors:
Model complexity: Larger models with more parameters require more computations, leading to higher energy consumption. For example, models like GPT 3, with 175 billion parameters, are significantly more energy-intensive than smaller models.
Data processing: The energy required to preprocess and clean data before it can be used for training is often overlooked. While this step is crucial for model accuracy, it adds to the overall environmental cost.
Training iterations: The number of training iterations or epochs directly correlates with energy use. Models that require extensive training cycles, especially those needing hyperparameter tuning, contribute more to carbon emissions.
Hardware efficiency: The type of hardware used, such as GPUs and TPUs, plays a significant role in energy consumption. While newer hardware is often more efficient, the overall environmental impact can still be substantial.
The urgent need for sustainable AI practices
As AI continues to grow in importance, there is a pressing need to adopt sustainable practices to mitigate its environmental impact. Here are some strategies that organizations can consider:
Optimizing model training: Reducing the number of parameters in a model without compromising performance can significantly decrease energy consumption. Techniques such as model pruning, quantization, and knowledge distillation can help.
Using green data centers: Investing in energy-efficient data centers powered by renewable energy sources can help reduce AI's carbon footprint. Companies like Google and Microsoft have already made strides in this area by committing to using 100% renewable energy for their data centers.
Prioritizing model efficiency over size: Bigger is not always better. Prioritizing model efficiency and performance over sheer size can lead to more sustainable AI practices. Researchers and developers should focus on creating models that are not only powerful but also environmentally conscious.
Implementing carbon offsetting: For unavoidable emissions, companies can invest in carbon offsetting projects, such as reforestation or renewable energy initiatives, to balance out their environmental impact.
The conversation around AI must evolve beyond its capabilities to include its environmental responsibilities. As the tech industry has embraced cybersecurity and data privacy, sustainability must become a core consideration in AI development. Companies that lead in this area will contribute to a healthier planet and gain a competitive edge as consumers and investors increasingly prioritize environmental responsibility.
The unseen costs of AI, particularly its carbon footprint, are a growing concern that cannot be ignored. As machine learning models become more complex and widespread, their environmental impact will only increase. By adopting sustainable practices now, the tech industry can mitigate these effects and lead the way in responsible AI development.
About the Author
Phanii Pydimarri, is the Data & Analytics Transformation Leader at Health Care Service Corporation (HCSC). Pydimarri joined HCSC in 2023 to lead data & analytics’ strategy, planning and strategic partnerships as part of the new Strategic Initiatives & Partnerships team.
In this role, he leads the development data, analytics, & AI strategy, and enabling strategic partnerships for HCSC’s Data & Analytics org aimed at external entities whose data, analytics, and AI capabilities support fast tracking transformative innovation, achieving rapid scalability, and competitive advantage thereby amplifying HCSC’s impact as an industry leader.
Before joining the company, Pydimarri was the Head of Commercial Products, AI & Advanced Analytics at Stanley Black & Decker, Inc. He also held several leadership roles at Bose Corporation, Sabre Corporation, Dallas Area Rapid Transit (DART), and SunGard/Capco Consulting Services. Hei holds master’s degrees from Northwestern University’s Kellogg School of Management and the New Jersey Institute of Technology.