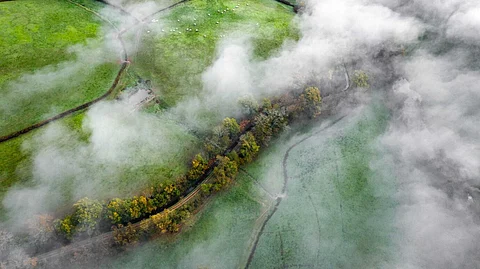
Google has introduced Scalable Ensemble Envelope Diffusion Sampler (SEEDS), a generative AI model that produces weather forecast ensembles at a fraction of the computational cost of traditional methods. Based on probabilistic diffusion models, it holds promise for weather and climate science applications.
How does Google's SEEDS work?
SEEDS, based on denoising diffusion probabilistic models, overcomes these limitations. It generates large ensembles efficiently, even from a few initial forecasts, matching or surpassing traditional ensembles' accuracy metrics. It provides more accurate likelihoods for extreme weather events while maintaining low computational costs.
It generates realistic weather forecasts by capturing spatial correlations and detailed structures, contrasting with traditional models that overlook correlations, hindering accurate assessments of weather phenomena, such as heatwaves.
Additionally, it also improves statistical coverage of extreme events, as demonstrated during the 2022 European heatwave. It extrapolates from seeding forecasts to provide better coverage and probability estimates for rare events, essential for emergency management and other application.
The Need for Probabilistic Forecasts: Chaos Theory and the Butterfly Effect
Weather forecasting faces challenges due to chaotic behavior, famously illustrated by the butterfly effect. Errors in initial conditions grow exponentially, limiting deterministic predictions. Probabilistic forecasts address this by generating ensembles, which quantify uncertainty. However, generating large ensembles is computationally demanding, restricting their usefulness for rare events.
Future Prospects
SEEDS showcases the potential of generative AI in weather forecasting, offering comparable accuracy to traditional methods but at a fraction of the computational cost. This hybrid approach, combining physics-based models with AI, promises advancements in weather prediction and allocation of computational resources. SEEDS represents a step towards enhancing operational numerical weather prediction and could find applications in climate risk assessment and other fields.
Google has been investing in weather research for a while now, introducing innovations like MetNet-3 and GraphCast. Traditional methods for weather forecasting rely on physics-based simulations to generate forecasts, but they are computationally expensive, particularly for rare and extreme events.