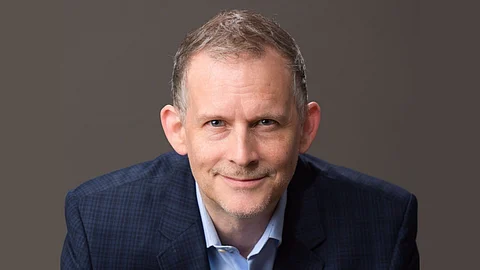
A hybrid cloud is a compute and storage environment where data and applications operate over both cloud and on-premises data centers (sometimes dubbed private clouds). Hybrid combines the benefits of on-demand unlimited scale with data center privacy and security (or more accurately, let’s face it: CDAO peace of mind). Hybrid offers cost-effective options for global enterprises which have data everywhere, and who must weigh options around performance, resilience, regionality, and corporate ESG objectives.
Hybrid clouds are proliferating because they bridge public cloud-native generative AI (GenAI) models with the private cloud data on which they must be trained. For massive, complex data estates, hybrid architectures are necessary to bring AI models to the data where it safely resides.
But simply adopting a hybrid architecture is not the answer to the problem here. We’ve been burned before by mandates that afforded insufficient cycles to vet the tech. That’s why discerning technology leaders are prudently seeking true hybrid solutions to avoid repeating the pain they experienced migrating to cloud — public cloud services are neither integrated nor compatible with private cloud services. Applications must be rewritten to operate across environments, and workloads must be painstakingly optimized to run affordably.
But what makes a hybrid solution true hybrid? There are at least 5 differentiators.
1. Portable interoperable data services: Production-scale AI applications often require the integration of numerous data sources which introduces complexity into a singular environment, more so in a hybrid environment. If simplicity is the art of maximizing the work not done, it is key to deploy workloads once and run them anywhere without having to rewrite them.
Public cloud migration was painful for many because workloads needed to be rewritten for cloud and then rewritten again in order to optimize them for affordability. It was so painful, in fact, that it precluded multi-cloud expansion.
True hybrid solutions provide portability and interoperability between environments enabling workloads to move friction-free and multi-directionally on any public cloud or on-premises in order to avoid refactoring costs. All of which will lower data service licensing costs.
2. Open data lakehouses: Equally as essential as the interoperability of data services, is leveraging true hybrid solutions that manage and transform massive quantities of structured and unstructured data on and between open data lakehouses: Unified platforms for data collection, storage, processing, and analysis.
Open data lakehouses preserve the flexibility of a data lake, and their data-science-at-your-fingertips design, while delivering the performance and domain-specific applications of data warehouses.
Connecting lakehouses via true hybrid enables the consolidation of ETL processes and the archival of duplicative datasets thereby reducing the data footprint and lowering cost. More importantly, reducing contradictory data leads to data asset standardization, unified data interpretation, and Utopia — trusted data!
3. Central command and control: Open data lakehouses afford a centralized vantage point from which to monitor and control the entire data estate, and with the right tools they can simplify it, even if data product ownership is dispersed throughout the organization in a data mesh.
True hybrid data platforms provide a common control plane with a single view to track cluster utilization and performance and pinpoint opportunities to reduce cloud costs.
4. Cross-platform security and governance: The common control plane also enables enterprise-grade data security and data governance which will be needed because multi-directional data flows and broadened data democratization increase the risk of data exposure and non-compliance.
Effective data governance programs are notoriously difficult and elusive in disconnected environments, and they are still ambitious propositions and multi-year journeys on integrated platforms. Therefore, true hybrid platforms must provide automation and tools to generate enterprise data catalogs, chart data lineage, and manage metadata.
5. Full data life cycle management: To maximize the efficiency of interoperability, true hybrid must offer a full data lifecycle toolset. According to Forrester, 75% of data practitioners and decision-makers reported they can save more than 4 hours each day if data lifecycle stages are integrated into a single platform.
Data collection and integration: Leverage streaming data services like Kafka and NiFi to collect and integrate real-time data for reporting and analytics.
Data storage: The secret sauce of open data lakehouses and an authentic game-changer, Apache Iceberg is a cloud-native, high-performance open table format that organizes petabyte-scale analytic datasets on a file system or object store.
Data processing: Table stakes for any platform.
Data analysis, visualization, and AI: In a recent study commissioned by Cloudera, “an overwhelming 90% of all respondents agreed that unifying the data lifecycle on a single platform is critical for analytics and AI. Nearly all perform fundamental data tasks such as ingestion, monitoring, and data pipeline processing, and 97% use traditional business intelligence tools.”
Anything less than true hybrid necessitates unacceptable recoding and reintegration, and with the pace of the race being dictated by the bullwhip of generative AI, we just haven’t got the time.
About the Author:
Shayde Christian is Chief Data and Analytics Officer at Cloudera. He guides data-driven cultural change for Cloudera to generate maximum value from data. Christian enables customers to get the absolute best from their Cloudera products such that they can generate high-value use cases for competitive advantage.
Previously a principal consultant, Christian formulated data strategy for Fortune 500 clients and designed, constructed, or turned around failing enterprise information management organizations. He enjoys laughter and is often the cause of it.