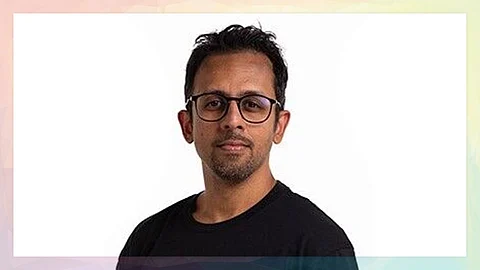
Analytics leader Sid Raisoni speaks with Savio Rodrigues, VP Client Partnership, Trianz – USA, about demand sensing as a solution to business problems and the four challenging pillars that must be considered while building data and analytic strategies.
Raisoni has held roles as Vice President of Analytics at WWE, Head of Analytics at Nestle Waters, and advises clients on the role of data through the lens of digital transformation.
He begins the conversation by shedding light on demand sensing. To explain its usage, he refers to a business problem of enduring high rates of lost sales due to product stock out and how demand sensing can come to the rescue.
To solve the problem, demand sensing can leverage data science to build machine learning ensemble models, to forecast price optimization and suggested orders, says Raisoni. He adds that this can be done at the SKU and customer level and can maximize both the supply chain and the demand side.
Delving further, Raisoni cites a more traditional business problem of forecasting deviations above industry averages, which increased during the pandemic because of volatility.
To tackle this problem, demand sensing deploys some of the latest machine learning demand forecasting algorithms, which are closely aligned to business levers, he asserts.
Being aligned with business levers it explains what the business can do about the problem, says Raisoni. This also brings transparency to why the forecast is telling what it is telling and enables the business to act a certain way. He adds that, unlike traditional approaches, demand sensing showcases the explainability of AI.
"We build AI solutions that predict demand at a granular level."
Sid Raisoni | Analytics Leader
Additionally, Raisoni mentions that he builds AI solutions for retail consumer packaged goods (CPG) and direct-to-consumer (D2C) companies, that predict demand at a granular level and provide business value by aligning leaders to proactively react to it.
When asked about the major challenges in implementing data strategies, Raisoni explains that he is passionate about the topic of data and analytics strategy and its role in organizational evolution and disruption of its ways of working.
In addition, he emphasizes the importance of approaching it from a holistic perspective and describes four dimensions that must be considered, including governance, technology, operating model, and culture.
Highlighting culture, Raisoni shares that it has a crucial role which encompasses systems, decisions, and norms that influence everything from hiring to training to incentives. He recommends addressing all four pillars for having a holistic strategy.
Delving deeper into the operating model of the analytics team, Raisoni asserts the need to think about the role of IT and business leaders and understand what they are looking for. He says that the goal is to change the way businesses operate by figuring out a way to have different teams work together to deliver new services.
Therefore, Raisoni says that building an operating model is not trivial, as it requires co-creation, a mindset, and a patience level that most people do not have.
Taking this point further, he maintains that things move slowly for people working in large companies, however, the change is massive once successful. Whereas, while one can be more agile and move faster while working for a smaller digital-native company, the impact will be smaller, concludes Raisoni.
CDO Magazine appreciates Sid Raisoni for sharing his insights with our global community.