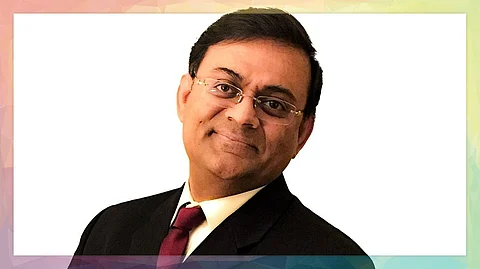
Enterprises typically exercise caution when adopting new technology, waiting until the technology matures and the vendor landscape stabilizes. However, generative AI is altering this paradigm. Following the excitement generated after the release of ChatGPT, enterprises are primed to explore the world of generative AI, aiming for tangible outcomes, productivity gains, and faster results.
The initial approach and strategy will vary across companies and even within different divisions of an organization. CEOs hold the key role in this decision-making process. They will play a pivotal role in deciding whether their company should pursue large-scale deployments or initiate smaller-scale pilot projects.
Another key factor is to identify the ‘right’ use cases – those that will empower organizations to move ahead at the required speed and achieve their business goals. However, it is essential to recognize that effective implementation of generative AI is not without its complexities; it demands a thoughtful and strategic approach.
Generative AI is a powerful technology that allows users to quickly generate new responses based on various types of inputs, including text, images, sounds, animations, 3D models, and more. This capability is driven by Large Language Models (LLMs) and advanced machine learning models that specialize in understanding and generating natural language responses and providing contextually relevant answers.
To achieve this proficiency, these models undergo extensive training on massive language datasets, which enables them to grasp the intricacies of language structures and meanings effectively.
However, while generic LLMs (often referred to as foundation models) serve as a solid starting point, they have limitations when it comes to addressing specific business needs. Each organization has its unique industry expertise, historical data, and operational nuances that cannot be fully captured by these one-size-fits-all models.
Generic LLMs lack the customization required to navigate and handle the unique intricacies of individual businesses. This limitation can result in suboptimal performance and only marginal enhancements in customer experiences. In essence, the convenience and speed of using generic LLMs may come at the expense of the control and customization necessary to meet specific business requirements.
On the opposite end of the spectrum, businesses have the option to construct their custom models using their enterprise-level data, thereby ensuring complete autonomy. However, developing a proprietary LLM is an arduous and resource-intensive task, demanding substantial investments of time, costs, and expertise.
Training large models requires access to extensive datasets and specialized infrastructure, which can be challenging for many companies. The costs associated with building and maintaining such models make it an impractical option for most organizations, especially in the initial stages of AI adoption.
For businesses looking to harness the full potential of AI models, especially when addressing the challenges of developing custom solutions, fine-tuning emerges as a highly recommended approach. Fine-tuning involves tweaking the base model's parameters to tailor it for performing a specific task.
In practical terms, this means refining the internal settings and features of the base model to better align with the nuances and requirements of the organization.
This approach not only enables organizations to capitalize on the capabilities of the foundation models but also allows them to leverage their specialized data, ultimately leading to enhanced performance. Fine-tuning provides a valuable degree of flexibility in selecting and refining models, giving businesses the ability to pivot as necessary based on continuous monitoring and evaluation.
For instance, consider a healthcare organization looking to deploy an AI model for medical image analysis. Fine-tuning allows them to adapt a pre-trained image recognition model to their specific dataset, thus tailoring it to recognize unique medical conditions and anomalies effectively.
This approach empowers CEOs and decision-makers to strike a balance between customization and efficiency while maintaining control and cost-effectiveness. Looking ahead, companies that can adeptly fine-tune AI models within their unique systems will not only differentiate themselves but also maximize the returns on their AI investments.
In essence, fine-tuning is the pathway to unlocking the full potential of AI in diverse industries and use cases.
To successfully implement generative AI at scale, organizations must establish a robust framework that provides them with a range of resources, such as solution approaches, accelerators, responsible design frameworks, and industry-specific solutions.
The journey begins when organizations identify the right business use cases where generative AI can deliver tangible value. These could range from streamlining repetitive tasks to delivering personalized customer experiences or enhancing product development.
Once these use cases are identified, organizations should assemble a cross-functional team comprising AI specialists, data scientists, and domain-specific experts. This diverse team will drive the integration efforts, ensuring that the deployment aligns seamlessly with the organization's strategic goals.
Emphasizing data privacy, security, fairness, compliance, and governance is also paramount. This involves the responsible and transparent management of customer data and AI-generated content.
CEOs must shoulder the responsibility of developing an ethical AI culture within their organizations. Investing in continuous training and development initiatives to upskill the workforce in AI technologies is crucial. Fostering an environment of innovation and experimentation will enable employees to harness generative AI's capabilities effectively.
The final frontier is to establish clear metrics and Key Performance Indicators (KPIs) to gauge the impact of generative AI on business outcomes. This data-driven approach will allow organizations to subject generative AI models to rigorous testing, continuously fine-tuning and enhancing them.
By following such a framework, organizations can navigate the integration process successfully and position their businesses for sustained growth and innovation.
Generative AI brings about unprecedented opportunities alongside unknown territories, compelling CEOs and business leaders to lead and take charge in a space that might feel unfamiliar and new. To drive substantial results, organizations should prioritize the right applications, invest in workforce development, and define clear success benchmarks.
Organizations that are ready to innovate their business models and ensure that generative AI experiments maintain ethical and secure practices can secure a lasting competitive advantage.
About the Author:
In his role as Co-founder and CEO for Innover, Amit Gautam is responsible for all aspects of the company’s product and services strategy and execution, as well as its financial performance and growth.
Prior to Innover, Gautam worked with firms like GE and Cognizant in various leadership roles. He studied Data Science at Harvard and holds a Bachelor's degree in Engineering from India.