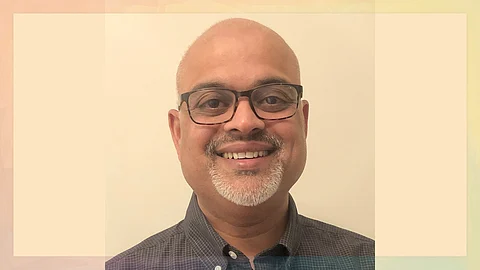
The developments in how we create, capture, process, harness, and manage data over the past few decades have been truly breathtaking. How data pervasively powers today’s businesses and all our lives is no less impactful than the advent of electricity and its impact on powering humanity.
This article is intended for CDOs, Data Managers, and Entrepreneurs to track the progression of data tech in line with developments in enterprise needs.
While core innovation in technology is always inspiring, looking at what the business needs today, tomorrow, and the day after helps evaluate new data tech opportunities. This article will discuss the relevant enterprise needs, and draw out core themes of data technologies that have the greatest potential to be desirable, viable, and feasible in the next 3-10 years.
Data has unmistakably become the primary fuel for modern enterprises to remain relevant and profitable. Unlike in the past, “data and data management” isn’t just another software implementation project. Data and the technology that allows the creation, capture, manipulation, analysis, and productization of data form the central nervous systems of the modern enterprise.
I call this suite of data infrastructure, platforms, and software services Data Tech. Data Tech covers the gamut across the lifecycle of data capture or creation, data movement, data storage, data vending and consumption, and archival.
Conventional data management and data tech patterns addressed 3-5 needs in an enterprise
Transaction support
Operational integration and reporting
Warehousing
Business intelligence, reporting, and analytics
The new stuff, the next big thing in Data Tech, will revolve around a different set of higher-order business concepts that are imperative for modern businesses
Speed to value
Capability cost parity
Employee experience
Consumer/customer experience
Compliance and regulatory
Speed to value:
Modern enterprises demand agility and speed to impact (not just software releases!). One cannot meaningfully iterate on products if there is no viable measurement of product performance, cost, and returns. Data Tech must enable quick and confident business decisions by being efficient in the capture, movement, access, and analysis of data across the enterprise.
A question for CxOs evaluating Data Tech to consider is, “What needs to be done during design time that generates value in runtime for enterprises?”
Capability cost parity:
Data Tech that allows businesses to operate, innovate, and iterate at a cost footprint comparable to their competitors is important. CDO’s costs for data management must be in line with that of other CDOs and across industry verticals.
Data Tech that is all about ‘capability’ without a comparative cost dimension (or net ROI dimension) is going to struggle. A key question to consider here is, “What is my cost of alternative choices vs the total cost of the data stack to achieve value?”
Human capital and employee experience:
Employees are at a premium, and their experience is paramount in recruitment and retention. Inspiration no longer comes from the “why” and “what” we ask our teams to work on. “How” they work plays a big role in employee experience.
Data Tech that understands the workday of a data engineer and delivers better employee experiences will be valuable. A key question to consider under this dimension is, “Can this data tech help me recruit better and offer a high-quality work environment for both data builders and data consumers?”
Consumer/customer experience:
Data Tech is the lynchpin for consumer experience. Live integration of consumer’s context, intent, and data is imperative in delivering the consumer experience of tomorrow. Data Tech that brings innovation and efficiency in areas of live data integration, inline security, and always-on intelligence will deliver superior consumer experiences – and therefore, will be successful.
A key question to consider under this dimension is, “How does this data tech power my customer experience?”
Always compliant:
Not an afterthought, and certainly not a quarterly/annual SOC2 time consideration, enterprises are required to stay current on compliance, and therefore, an imperative for Data Tech. Continuous compliance from data systems is a “thing” – and not a bolt-on from a Cyber or a Risk management vendor.
A key question for CxOs evaluating Data Tech to consider under this dimension is, “What out-of-box capabilities and features does this data tech offer to help me with my continuous compliance goals, and does this play nice with the rest of my enterprise risk management stack?”
To deliver on the business needs, a set of core technology underpinnings is necessary for the new Data Tech:
Reliable delivery and resilient ops
Live data integration
LLMs and always-on AI
Continuous compliance
Data products
Reliable delivery and resilient ops:
Next-gen Data Tech must facilitate iterative delivery of consistent and ‘reliable’ streams of capability to the enterprise. It must fit the ‘agile’ operating model and fully integrate with the rest of the enterprise development methodology.
Rapidly embracing the no-code/low-code genre is desirable and must permeate data tech as well. This agile and reliable delivery Data Tech must offer an integrated observability feature set that works with the broader enterprise. Finally, while resilience is table stakes, unhinged elasticity, and resilience cost footprints are not acceptable to enterprises anymore.
Data Tech will need to integrate optimized elasticity and resilience where service levels and failover conditions are fully met while intelligently forecasting and scaling to keep the costs under control.
Live data integration:
Data Tech of the future must continue to drive innovation within streaming by way of stream analytics, stream integration, and stream management. Enterprises will need Data Tech to enable real-time integration of consumer’s device/location data, enterprise CRM data, pricing, and offer engines, competitors’ price matching and calibration, supply-chain data, and financial models to create solutions and experiences that match the next-gen consumers’ expectation of live and concurrent service.
Data Tech that exploits the power of parallel processing offered by hardware innovation like those of GPU-based computing (as opposed to general-purpose computing CPU powerhouses of the past) will be critical in this area of live integration and performant data processing.
LLMs, always-on AI and analytics:
Our industry has seen some strong innovations in search-based data/reporting solutions. Large language models (LLMs) are resetting expectations of user interfaces with a business. Next-gen Data Tech will require LLM-based interfaces to democratize access to data in previously unimaginable ways.
Next-gen Data Tech will have to help enterprise CxOs solve both Boolean problems (problems with one of two results: true or false) (as in traditional business intelligence reports) as well as probabilistic problems that provide predictive capabilities for enterprises to proactively capitalize on opportunities and mitigate risks.
A combination of algorithmic AI/ML with the generative capabilities of LLMs – creates this “Always-On AI” confluence – that then will transform enterprise and their customer interaction with each other, and their data. AI and LLMs cannot remain a beta/R&D exercise, they must come into the mainstream with a real ROI – and next-gen data tech has to enable that elusive ROI.
Continuous compliance (devSecFinGovOps):
With Cloud-based IaaS/PaaS solutions, Microservices architectures, and CICD delivering enterprise value in terms of agility and flexibility, the need to manage the rest of the enterprise’s constraints in terms of security, cost, and compliance, are now at the fore.
New Data Tech must support encryption, tokenization, and additional sophisticated forms of synthetic key & hash management – and be able to offer businesses the capability to process such secured data and generate meaningful insights. While Data Tech must enable rapid ingress of data from ever-emerging and diverse sources, Data Tech must also help the CDOs manage the quality, provenance, and proliferation.
Instead of ‘OTT’ style lineage and quality products that ride on top of existing data stores, in-line lineage and DQ products that can establish provenance and quality parameters while also facilitating performant movement of data will create value for enterprises.
Data and Data Products as a Service (DaaS, DPaaS):
The ability of Data Tech to help enterprises drive DaaS and DPaaS agenda is a higher-order value prop, and therefore of higher value point. Data Tech that allows enterprises to rapidly identify the uniqueness of their data sets, bundle them with advanced analytics – and abstract those analytics as products that other ecosystem participants can run will command a premium in the market.
The DaaS and DPaaS strategy need not be externalized products and services, these capabilities could serve internal customers just as well. Data Tech that enable companies to visualize products and rapidly vend them as services will win big in the enterprise markets down the road; much like the way business intelligence used to help enterprises visualize trends in data.
Nothing is more fascinating in our data tech world today than the many lucid and yet confounding patterns of descriptions, applications, and patterns on data fabrics and data mesh. Data fabric is principally a technology construct, while data mesh is an organizational construct that encompasses people-process-and-technology in delivering data products and services.
Fabrics evolve from data virtualization underpinnings and solve for unavoidable fragmentation of data stores in an enterprise. Data architectures that follow domain-centric disciplines tend to have data stores by business unit/domain – customer, product, procurement, etc.
A similar data store oligarchy would exist in data architectures that are more functional – data stores across transactional systems, operational integration stores, warehouses, purpose-built appliances and marts, cloud lakes, etc. The net result is disparate stores that primarily serve the domains they are built for, and secondary sustain integration use-cases across domains.
Fabrics are powerful in solving this problem by creating a self-serve framework that supports data discovery, data provisioning, data access and consumption, and does so virtually. Data tech that supports or enables fabric architectures will drive positive business impact in the future.
Data mesh on the other hand is an integrated operating model concept of how people-process-and-tech come together to create a solution space. Data mesh concepts emphasize ownership of data either by domain, use-case, or other organizational variants, and affect a product outcome – where data is managed like a product.
Data product managers undertake the same responsibilities as any other product managers in the enterprise in scoping/defining the market (internal or external), conducting CX/UX research, driving a data product roadmap, measuring product adoption and performance as well as P&L (direct or indirect).
Data mesh is to traditional monolithic databases as are microservices to traditional monolithic applications and systems. Data mesh is the true collision of lean-agile and product-centric thinking making its way into data.
Fabrics create a virtual centralization of data in an enterprise in support of business agility, while meshes support business agility through a hyper-efficient data-as-a-product model. Both will serve to get the data a business needs but in different ways.
For data tech vendors and enterprise leaders, the key is to look for products that have data fabric tech capabilities, and a build-deploy-operate model that supports agile data-as-a-product delivery model.
Architecture and accountability:
Key to data organization of the future is the integration and evolution of the traditional enterprise architect with that of a conventional data architect that optimizes data IaaS, PaaS, and SaaS choices based on a unified technology strategy that enables a participatory and shared data economy within an enterprise.
New data tech that capitalizes on this trend by embracing open architectures and fosters cross-enterprise applicability will do better than purpose-built, bespoke solutions (aka islands of good!).
The rapid innovation in data tech over the past decade, especially with big data, AI, ML, and now LLMs – has created a large wave of R&D programs in enterprises driving innovation, education, and encouraging the adoption of new technologies and solutions.
These R&D waves or innovation labs will have to converge into mainstream IT accountability by demonstrating true business lift. Emergent data tech must enable business units and data R&D functions alike to realize a return on their investment quickly to belong in the enterprise stack.
Conclusion
Data Tech remains one of the most promising tech sectors in current times. Cyber will probably stay ahead given the risk/liability associated with that enterprise need, but Data Tech will remain the nervous system of the next-generation enterprises.
We discussed the business needs of the next-gen enterprise, with cost parity, speed to value, customer experience, employee experience, and continuous compliance.
We then looked at the tech capabilities needed to help enterprises realize their goals by way of providing reliable and resilient solutions, live data integration and analytics, always on AI, continuous compliance, and DaaS/DPaaS. Many of the predictions we have are in development and various stages of maturity.
However, for an enterprise CDO, a Data Tech investor, or a Data Tech entrepreneur it is imperative to see the enterprise needs as the primary indicator of success for new Data Tech.
Acknowledgements:
Mark Settle. Author, Coach, Mentor, Veteran CIO & Industry Thought Leader
Kumar Bhogaraju, Ex-VP Engineering and India Site-Lead Groupon Labs
Satya Addagarla, CIO, Wells Fargo Home Lending
Vijay Anisetti, Senior Software Engineer MentorGraphics, Ex-Senior Technical Yahoo at Yahoo, Ex-Engineer Informix
Saqib Awan, General Partner, GTM-Capital
About the Author:
Prabhakar Bhogaraju (PB) has been a data, product and technology practitioner, architect, manager, and executive for over 25 years. He has deep expertise in the application of data, analytics, and AI tech in the housing finance industry, and has held multiple executive leadership positions in product development, data management, and digital business architecture at Fannie Mae.
He is also active in the venture and startup ecosystems, served in investment councils and expert committees for enterprise tech focused venture funds, as well led strategy, product, and development functions for a FinTech startup.
Bhogaraju is an author and speaker in various industry forums on emerging technology applications for the housing industry. He founded an emerging technology advisory firm ALE-Advisors, and is a limited partner with GTM-Capital, a member of the Board of Advisors for the Research Institute for Housing America and Chair of the Certified Mortgage Bankers Technology Committee of the Mortgage Bankers Association.