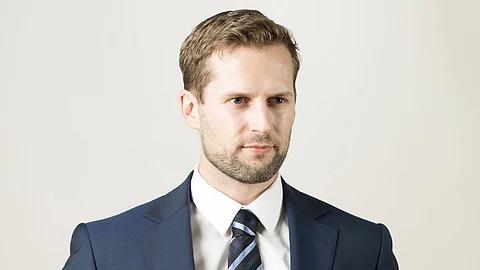
Simon Nuss, VP of Data and Analytics, Hitachi Solutions, Canada, speaks with Jason Brandt, Managing Partner and Commercial Officer at Stagwell Technologies, in a video interview about creating a successful analytics department, the need for measuring performance, aligning the data team with the available tools, the choice between source vs managed tools, and the critical factors for managing data.
According to Nuss, creating a highly functional analytics department has the following prerequisites:
Hiring strong talent: Not hiring the right talent will have future ramifications. Instead of settling for “good enough,” leaders should invest time and pursue excellent people.
Champion them within the organization: Establish their brand and identity not only amongst themselves but also the organization. Let them establish their brand and host social or community events. Give them the confidence they need to approach the business and keep going.
Measuring the department every week/day: Document all the activities like POCs, meetings with the business, workshops, certifications, projects completed, and value brought to the business. That report will be important during budget discussions.
Champion leaders: It will attract talent to the organization and other employees will want to upskill themselves to meet that threshold.
When asked if skills are keeping up with the available tools, Nuss says that while the data engineering pipeline hasn't changed very much, the number of tools has ballooned in two years.
He says that teams using open-source tool sets will face challenges as they are updated every two to three years on average and users have to adjust their skills to keep up. The challenge does not exist with managed solutions.
Elaborating on the approach to deciding what tools to use, Nuss mentions that legacy technology plays a key role here. Existing talents will have biases towards existing technology which will push the choices in a specific direction.
In the case of greenfield scenarios, it depends on the choice of data engineers between open-source and platform-focused options.
Nuss however stresses that users of open-source stacks must stay up to date on the latest developments and understand what is emerging and disappearing.
Speaking about the critical factors necessary to collect, store, catalog, secure, democratize, and operationalize data, Nuss mentions the following:
Data collection: Gathering data can be technically challenging, especially when dealing with data owners who may be hesitant to grant access or provide data in non-standard formats like CSV or Excel. Additionally, reliance on APIs from SaaS vendors can be problematic due to throttling or limited data availability.
Data storage: Storing data is critical, and many organizations opt for open file formats that can be queried by various engines. This approach enhances accessibility and flexibility, reducing dependence on specific vendors like Snowflake.
Data security: Data security is generally considered to be well-addressed, with measures such as firewalls, virtual network peering, robust authentication models, and strong governance around identity management. Various tools have also emerged to support security efforts.
Operationalization: The process of operationalizing data is relatively straightforward, but the challenge lies in encouraging people to use it effectively. A crucial success factor is to track and measure everything, ensuring that monitoring reports are in place to analyze customer usage patterns for operationalized data.
To simplify this further, Nuss emphasizes the importance of comprehensive measurement and monitoring practices.
CDO Magazine appreciates Simon Nuss for sharing his insights with our global community.